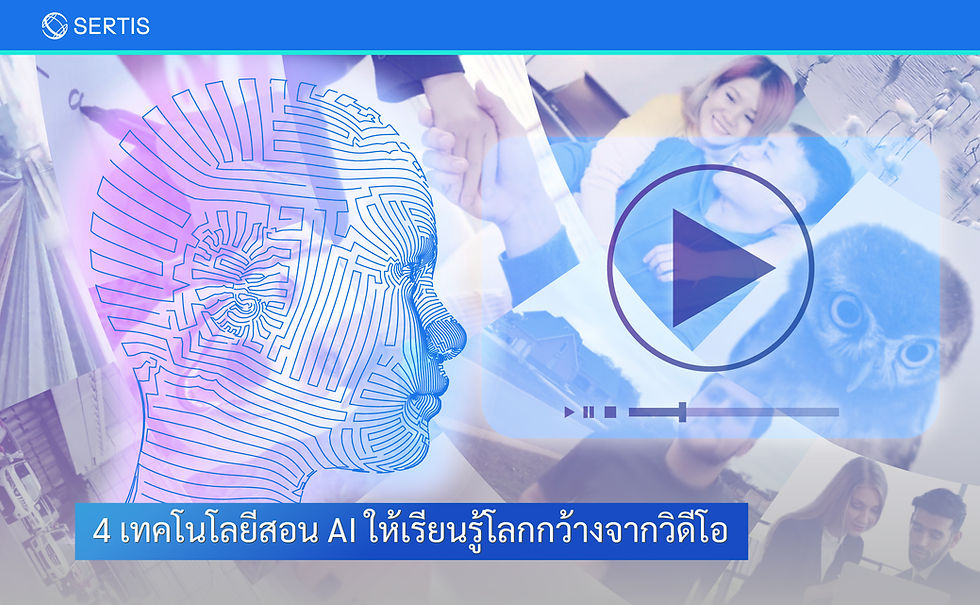
The ideal goal of developing AI is to have AI that can perfectly mimic the way humans think, learn, and make decisions. We, humans, learn automatically from the world around us. If AI can do the same, it will open rooms for more and more advanced innovations.
Nowadays, AI has come closer to that goal. Several tech companies have introduced technologies that enable AI to learn from video. Videos uploaded on social media represent events happening around the world. Learning from videos allows AI to learn like humans do, which develops AI to be more like humans.
Today, we would like to introduce you to 4 technologies that are an attempt to provide AI with
an ability to learn from video by using either datasets or a self-supervised learning technique.

The Moments in Time dataset by MIT-IBM Watson Lab
Even though there are datasets that teach AI to recognise actions in videos, they can only understand a specific action. AI can not explain the sub-actions that make up those actions. For humans, deconstructing actions in this way is just a piece of cake, but for AI, it has been a big challenge. For instance, AI knows the high jump but does not understand that a high jump consists of many basic actions, running, jumping, arching, falling, and landing. Using video snippets of labeled basic actions data, chosen to cover frequently-used English verbs, including sounds like clapping sounds, allows the development of multi-modal models.
This Moments in Time dataset can also recognise the same action in several different environments. For example, opening the door, opening the curtain, and the dog opens his mouth are all categorised to be ‘opening’ by using temporal-spatial transformation.
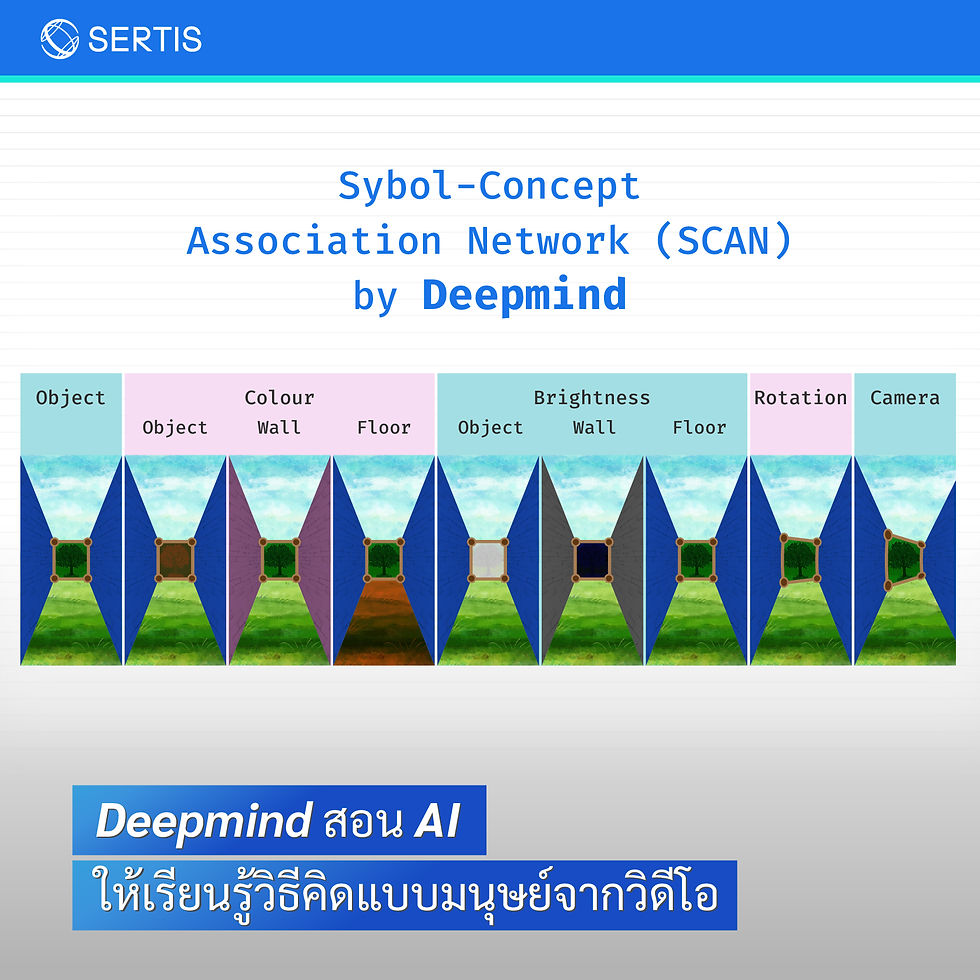
Symbol-Concept Association Network (SCAN)
In 2017, DeepMind had attempted to train AI to learn from videos by itself without any labeled data from humans. The developers use the same method as to how children learn about the world. The data used to train the model are the stills from the videos and 1-second audio clips from the same point of the still images in the video. SCAN algorithm consists of three separated neural networks for recognising images, recognising sounds, and comparing matching images to sounds. If the model found a picture of similar actions, it will pair them with what it has learned.
DeepMind also developed a neural network called Symbol-Concept Association Network (SCAN). The system can learn a new concept and combine it with something familiar. For example, how the system recognises apples is not by remembering the picture of apples and comparing it to other images. It understands the actual size, shape, and color of apples. When the system sees a photo of apples that is not the same as it saw before, it can automatically recognise them

CLEVRER and NS-DR System by IBM After recognising objects in video, another step of learning from videos is understanding the reasons and relation of objects and events. In the past, if you showed AI a video of a man hitting a ball with a bat and asked what would happen if he missed the ball or which direction the ball would go, AI would not be able to answer them. AI could only recognise the object but knew nothing about the motion, gravity, or impact. That was why it could not answer causal questions. Researchers from IBM, MIT, Harvard, and DeepMind have introduced a new dataset called Collision Events for Video Representation and Reasoning (CLEVRER) and a hybrid AI system, Neuro-Symbolic Dynamic Reasoning (NS-DR). CLEVRER consists of videos of objects moving and colliding with one another. What AI agent needs are abilities to recognise objects and events, model the dynamics and causal relations between the objects and events and understand the symbolic logic behind the questions The developers developed NS-DR because other models can not effectively use CLEVRER's limited and controlled environment and can handle causal and counterfactual scenarios. NS-DR is a combination of neural network and symbolic AI (rule-based AI), the old-fashioned AI with symbolic-reasoning ability. The neural network also works when the data is limited because it requires less data than other models. NS-DR bring out the strengths of both systems and can overcome the weakness of CLEVRER.
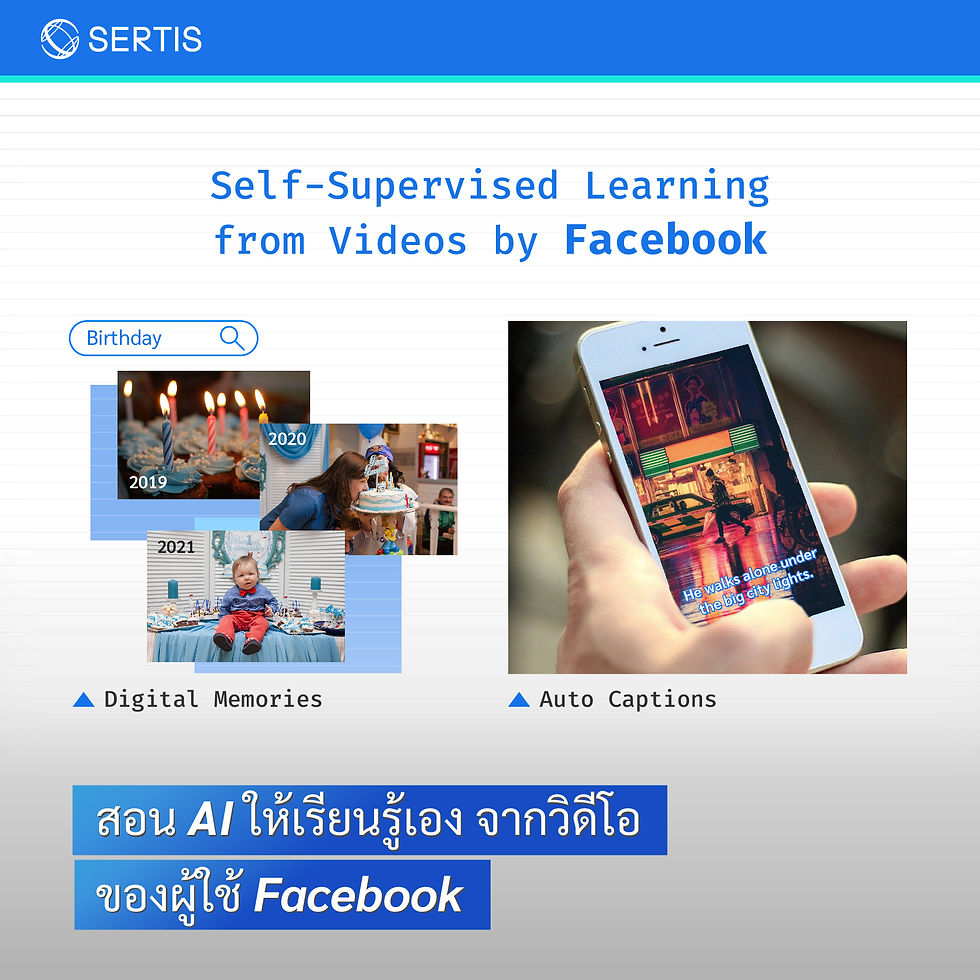
elf-Supervised Learning from Videos by Facebook
Facebook has just launched a new project called 'Learning from Videos', which created self-supervised learning AI that learns automatically from videos uploaded publicly on its platform. This technology overcame the obstacle that AI models need to use data labeled by humans and speeded up the training process. Also, it may deepen AI's ability to learn and analyse because it has to connect the dot by itself.
Videos uploaded by 2.8-billion Facebook users are culturally diverse and up to date. Training AI with these videos will result in adaptive AI that fits the fast-pacing world. Generalized Data Transformations (GDT) technique helps AI understand sounds and images in videos. The technology also improved speech recognition by using wav2vec 2.0. Facebook applied this technology to Instagram Story by adding the Auto Captions feature that automatically generates subtitles in our video. Facebook also used the technology with Instagram Reels recommendation systems (New Instagram feature that allows users to create short creative video clips like TikTok). The technology helps them find videos that are in the same them (same music, dance moves, or categories)
Another cutting-edge feature that Facebook has planned is Digital Memories. This feature allows us to find videos using only a keyword phrase, for example, Birthday Party. AI will go through every type of data and match ‘Birthday Party’ to people singing Happy Birthday songs, cakes, candles, or anything signifying a birthday party. Digital Memories feature is designed to be featured in smart eyeglasses, another big project that mainly facilitates people to capture and revisit the memories through their eyes.
References:
https://ai.facebook.com/blog/learning-from-videos-to-understand-the-world/
https://www.theverge.com/2021/3/12/22326975/facebook-training-ai-public-videos-digital-memories
https://www.newworldai.com/ibm-new-dataset-to-help-ai-with-video-understanding/
https://www.ibm.com/blogs/research/2017/12/ai-video-understanding/
https://bdtechtalks.com/2020/05/04/clevrer-dataset-ai-video-reasoning/
Commentaires